PPG2ECG两种算法比较
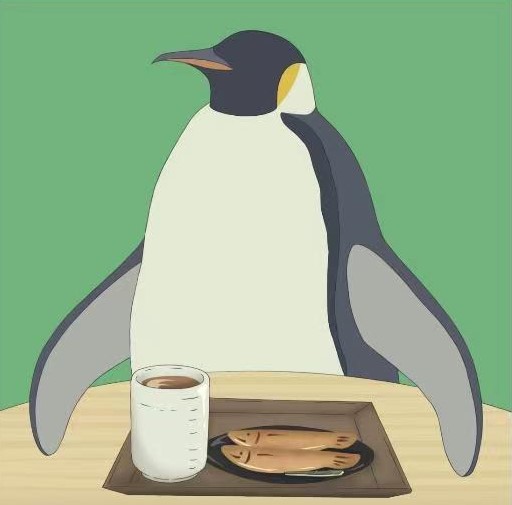
PPG2ECG两种算法比较
Lin 07/22
一些零碎的知识
.npy
文件
用于储存numpy数组的二进制文件,读取速度更快,占用内存更少。简单用法如下:
1 | test = np.load('文件名',encoding = "utf-8") |
PPG2ECG-WGAN
利用GAN来训练生成器
链接:https://dl.acm.org/doi/10.1145/3412841.3441979
Loss Function
为了使得GAN更加容易收敛,采用Wasserstein GAN模型,直接根据输出值大小评价效果,目标函数中也不需要对数
where
0 and 1:
functions. The gradient penalty is weighted by a factor
L2范数作为损失函数希望生成器在对抗判别器的时候尽可能接近真实的心电图数据
同时,为了使得ECG的特征更加明显,加入对Peak和Valley的距离损失函数
综合以上三个损失函数,可以得到该模型的目标损失函数
系统框架
decoder-encoder
评估指标
Pearson’s Correlation Coefficient
就是概统课上讲的相关系数,越接近于1越好
希望通过Pearson’s Correlation Coefficient来衡量具体的相关程度
Root Mean Squared Error
方均根误差,越小越好
Fréchet distance
这个就非常有意思了,为了衡量曲线和曲线之间的相似程度的一个指标。具体定义为:
复现过程
论文和github中都没有提到应该选用哪个python版本,查看服务器上
3.8.16
版本较多,遂选择了这个版本。使用Conda安装实验环境
1 | conda create -n P2EWGAN python=3.8.16 |
根据github上的步骤,安装所需环境
1 | pip install -r requirements.txt |
PPG to ECG TAAN
Transformed Attentional Neural Networks
https://ieeexplore.ieee.org/stamp/stamp.jsp?tp=&arnumber=9109576
框架
基于encoder-decoder使用CNN来实现ECG的重建
encoder-decoder network
- Sequence transformer network
- 可自动calibrate the offset
- Attention network
- identify regions of interest
- Proposed QRS complex-enhanced loss
思路
Seq. Transformer Network
CNN受到magnitude和phase的影响很大,所以需要先归一化(用一个Seq. Transformer Network)
(实际上就是进行了一个线性变换?)
先是时序变换
再是幅值变换
Attn.
用两个全连接层和softmax作为激活函数
Encoder-decoder
Loss Function
采用L1范数使得信号更加强烈
但是,单纯使用L1范数不能体现出QRS Complex中一些峰值的重要性,同时,特别是R峰,偏离一点就会导致L1范数巨大,所以需要重新设计损失函数。
Let
评估指标
- Normalized Mean Absolute
Error (NMAE) - Normalized Root Mean Square Error (NRMSE)
对于R peak区域,采用Mean Location Error(MLE)来衡量
Let
其中
对于R peak区域的幅值,可以用Mean Magnitude Error(MME) 来计算
复现过程
什么python=3.6 … 明明应该是3.8,不然好多好多包都安装不了
TODO
(GAN模型) pix2pix
CLIP和MAE(mask autoencoder)
- Title: PPG2ECG两种算法比较
- Author: Yuze-L
- Created at : 2024-07-22 21:01:15
- Updated at : 2024-07-31 14:54:47
- Link: https://yuze-l.github.io/2024/07/22/P2EWGAN/
- License: This work is licensed under CC BY-NC-SA 4.0.